The role of metrology in AI-enabled digital biomarker research and development
Keywords:
digital biomarkers, artificial intelligence, validation, data quality, healthcare technologyAbstract
The article explores the role of metrology in the research and development of digital biomarkers, particularly in the context of artificial intelligence and large language model integration. It highlights the lack of standardized evaluation frameworks as a major barrier to the clinical adoption of digital biomarkers. By introducing a metrology-driven approach, the study proposes systematic methods to ensure traceability, validation, and standardization, thereby fostering trust, interoperability, and industrial scalability of AI-enabled digital biomarker solutions in healthcare.
References
Coravos, A. et al. (2019). Digital biomarkers for health monitoring. NPJ Digital Medicine.. https://www.nature.com/articles/s41746-019-0090-4
FDA (2018). Bioanalytical Method Validation Guidance. https://www.fda.gov/media/70858/download
Powell, D. (2024). Walk, talk, think, see and feel: harnessing the power of digital biomarkers in healthcare. NPJ Digital Medicine. https://www.nature.com/articles/s41746-024-01023-w
Wilkinson, M. D. et al. (2016). The FAIR Guiding Principles for scientific data management and stewardship. Scientific Data. https://www.nature.com/articles/sdata201618
DiMe Society (2023). The Playbook: Digital Clinical Measures. https://playbook.dimesociety.org/
Pálhalmi, J. et al. (2019). A Statistical Metrology Approach to Compare the Quality of Optical Pulse Wave Signals. Computing in Cardiology , Vol 46. DOI: 10.22489/CinC.2019.165 https://www.analog.com/media/en/technical-documentation/tech-articles/optical-integration-without-compromises.pdf
Tretter, M. et al. (2025). AI-produced certainties in health care: current and future challenges. AI and Ethics. Springer Nature. https://link.springer.com/article/10.1007/s43681-023-00374-6
Bommasani, R. et al. (2022). On the Opportunities and Risks of Foundation Models. arXiv https://arxiv.org/pdf/2108.07258
Chen, S.-Y. et al. (2024). Perceptions of ChatGPT in healthcare: usefulness, trust, and risk. Frontiers in Public Health. https://www.frontiersin.org/journals/public-health/articles/10.3389/fpubh.2024.1457131/full
Open mHealth. https://www.openmhealth.org/
PhysioNet. https://physionet.org/
FDA Guidance on Clinical Trials. https://www.fda.gov/regulatory-information/search-fda-guidance-documents
EURAMET EMN for Health. https://www.euramet.org/metrology-for-societys-challenges/metrology-for-health
Mathews, S. C. et al. (2019). Digital health: a path to validation. NPJ Digital Medicine. https://pmc.ncbi.nlm.nih.gov/articles/PMC6550273/
Large Language Models for Medical Applications. Frontiers in Medicine. 2025. https://www.frontiersin.org/research-topics/64598/large-language-models-for-medical-applications
IMDRF SaMD Definitions.
https://www.imdrf.org/documents/software-medical-device-samd-key-definitions
European Medicines Agency:
Pálhalmi, J. et al. (2024). AI-Powered Microscopy Platform for Airborne Biothreat Detection. Springer Nature.
https://link.springer.com/chapter/10.1007/978-3-031-62083-6_10
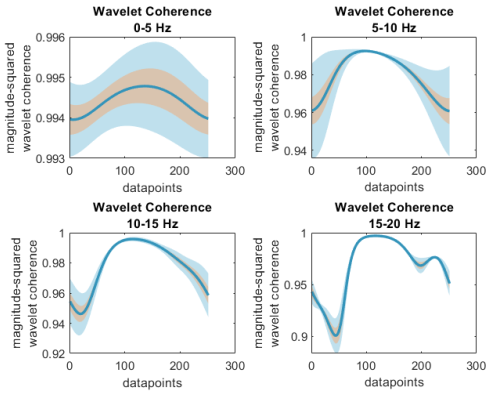
Downloads
Published
Issue
Section
License
Copyright (c) 2025 Bánki Közlemények (Bánki Reports)

This work is licensed under a Creative Commons Attribution-NonCommercial-NoDerivatives 4.0 International License.
Bánki Közlemények is loyal to open access for academic work. All the original articles and review papers published in this journal are free to access immediately from the date of publication. We don’t charge any fees for any reader to download articles and reviews for their own scholarly use.
The Bánki Közlemények also operates under the Creative Commons Licence CC-BY-NC-ND. This allows for the reproduction of articles, free of charge, for non-commercial use only and with the appropriate citation information. All authors publishing with the Bánki Közlemények accept these as the terms of publication.